Now 25 visitors
Today:268 Yesterday:500
Total: 38262
16S 4P 0R
2025-07-09, Week 28 |
TACT Journal Page |
Call for Paper |
Author Page |
Paper Procedure |
Paper Submission |
Registration |
Welcome Message |
Statistics |
Committee |
Paper Archives |
Outstanding Papers |
Author Page |
- Paper Procedure |
- Journal Procedure |
- Presentation Tips |
|
Proceedings |
Program with Papers |
Plenary Session |
Tutorial Session |
|
Presentation Assistant |
Hotel & Travel Info |
About Korea |
Accommodation |
Transportation |
VISA |
Other Infomation |
|
Photo Gallery |
FAQ |
Member Login |
Scheduler Login |
Seminar |
Archives Login |
|
Sponsors |
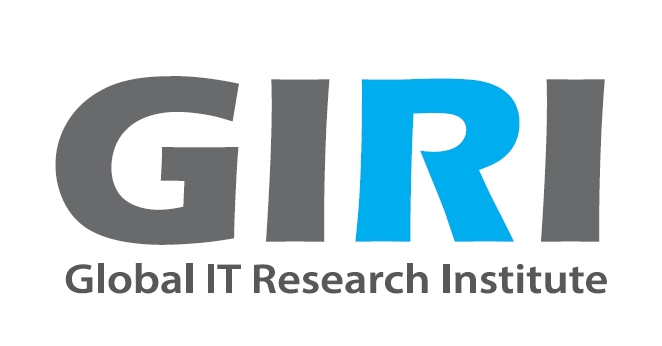
|
|
|
|
|
|
|
|
|
|
|
|
|
|
|
¨Ï Copyright 1999. ICACT (ISSN 1738-9445) & Global IT Research Institute(GiRI) All rights Reserved. Contact: office (at) icact . org Tel:+82-70-4146-4991
|
1713 Obelisk, 216 Seohyunno, Bundang-gu, Sungnam Kyunggi-do, Republic of Korea 13591
Business License Number : 220-82-07506, President: Thomas ByeongNam Yoon Ph.D. |
(13591)°æ±âµµ ¼º³²½Ã ºÐ´ç±¸ ¼Çö·Î 216 ¿Àº§¸®½ºÅ© 1713È£
(»ç)±Û·Î¹úIT¿¬±¸È¸ »ç¾÷ÀÚµî·Ï¹øÈ£ : 220-82-07506 ´ëÇ¥ÀÚ : À±º´³²
|
|
| |